AI in Radiology Today
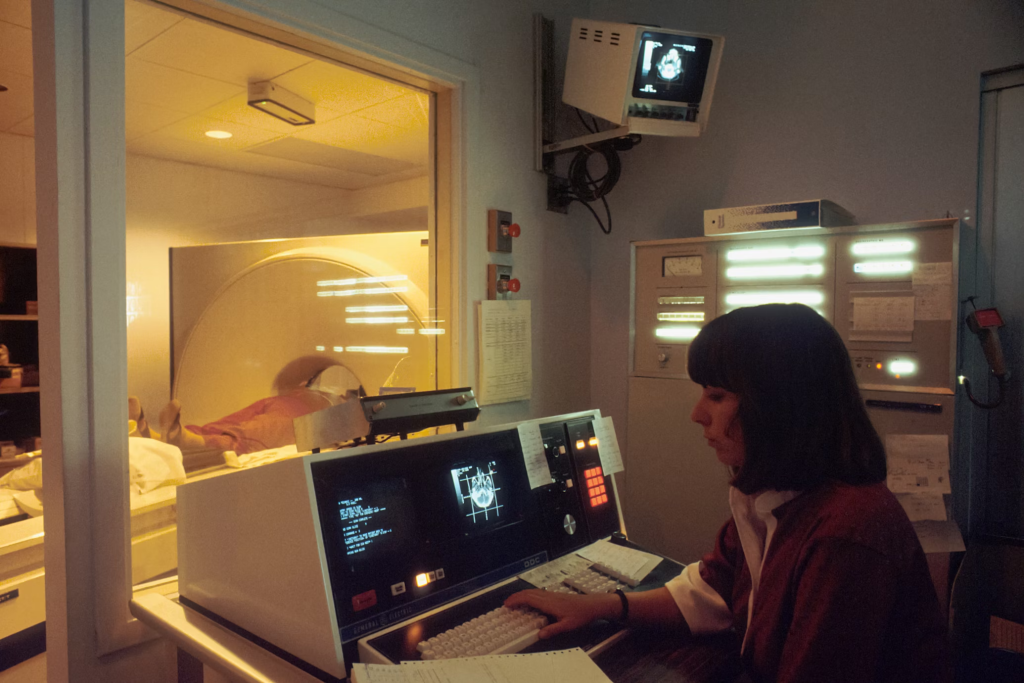
AI, a powerful tool that has had an impact on various careers, seems to be making its way towards many Radiologist’s Imaging rooms. AI algorithms are specifically being developed to assist Radiologists read data and to provide a much more accurate analysis. A recent Artificial Intelligence(AI) algorithm known as Rad AI for example, is an Artificial Intelligence based tool that can automate basic radiology tasks for Radiologists such as anomaly detection, generating reports, and pattern recognition. Other AI enterprises that provide Radiologists with assistance include NVIDIA Clara Imaging and IBM Watson Health.
The expectations on a daily basis of radiologists continues to increase exponentially as the rising number of medical imaging studies, the need for punctual and reliable diagnoses, the risk of human error, the growing demand for radiology services, etc. AI has been a great way to regulate all these issues within the healthcare field. AI algorithms are able to organize Radiologists workflow, therefore Radiologists can prioritize critical cases. AI tools are also able to identify abnormalities in medical images, minimizing the risks of misinterpretation through pointing out potential erratic or unusual findings in medical images. While being able to identify these anomalies, AI algorithms are faster than humans leading to rapid results and possibly quicker recovery. In regards to recovery, AI algorithms can provide possible treatment plans based on the patient's information to create personalized care for patients.
Regardless of its favorable results as it continues to grow, AI also holds its vital disadvantages to consider.The AI tool ChatGPT-4 made by Open AI, has been proven to be nothing short of excellent. ChatGPT-4 has been able to identify abnormalities in kidneys while providing additional facts concerning the abnormalities found through ultrasound images(Sultan, 2024). With 20 percent of radiologists planning to use it in the near future although it has just recently become popular.(Itransition, 2024). The John Hopkins hospital has formed a subcommittee called Radiology Artificial Intelligence Development (RAID). RAID’s main motive is to study the use of AI in radiology as well as the advantages that it brings. The RAID team continues to study AI in radiology because of the incredible abilities it has to make the workflow of radiologists easier, provide analysis faster and more efficiently, and also serves as a form of confirming or verifying analyses. With 400 radiology AI products on the market, which have also been cleared by the FDA (and that number increasing) it is needless to say that AI can take some of the responsibilities of many Radiology departments which ensures confidence in interpreting images. Furthermore, RAID also hopes to bring the incredible use of AI from the “bench to bedsides”(John Hopkins Radiology) . They hope to increase the use of AI within the radiology department through future advancements such as Radiology AI labs, as well as using AI in the John Hopkins radiology department. This is an innovative way to enhance assistance in classifying medical images with the help of AI. The immense work and dedication to incorporate AI into the daily lives of radiologists exhibits the vast improvements and upsides AI can bring.
Regardless of all these advantages and advancements, there are still challenges and questions surrounding the use of AI. The question being, really just how reliable is it? In research done by a team at the University of California Davis, it was found that although ChatGPT continuously answered over half of the 30 cardiac imaging questions correctly, at least one-fourth were inaccurate or “clinically misleading” (Clinical Imaging, 2024). Although ChatGPT-4 proved to provide more accurate results compared to ChatGPT-3.5, additional advancements in AI are needed to ensure an absolute accurate interpretation of data. Additional research from Duke University(Radiological Society of North America, 2024) depicts inaccurate conclusions by AI algorithms due to the characteristics of patients. An AI algorithm was given the task to analyze the breast imaging of 4855 women: 1316 white women, 1261 black women, 1351 asian women, and 927 hispanic women, with a median age of 54. Upon receipt of the results, it was found that 17% of the cases were cataloged as suspicious by the AI algorithm yet were classified as false positives/incorrectly identified as suspicious by the AI algorithm upon further analysis. This research proved most of the “suspicious” cases identified by the AI algorithm were the cases of women of color. In comparison with white patients, black patients were more likely to portray abnormal case scores according to the AI algorithm (odds ratio [OR] = 1.5 [95% CI: 1.2, 1.8]) and Asian patients were less likely to portray abnormal case scores (OR = 0.7 [95% CI: 0.5, 0.9]). In addition to race, age also had an impact on the AI algorithm’s results. It was found that younger patients ages 41-50 years were less likely to have false-positive results compared to older patients, ages 71-80 years old. The challenges behind the use of AI within radiology remain as there are factors contributing to its ineffectiveness at times causing it to not be one hundred percent accurate no matter the circumstances.
From X-Rays to MRI’s and CT scans to mammographies, and detecting other bodily complications, AI has been proven to be a very big help for many radiologists. This does not take away the fact that remains of its negative components of not being undoubtedly correct. AI is a powerful tool that can change the lives of many radiology professionals as long as it is used properly and handled well due to its limitations.
Work Cited
- Monroe, Cynthia L., et al. “Evaluation of responses to cardiac imaging questions by the artificial intelligence large language model ChatGPT.” Clinical Imaging.org, 23 May 2024, https://www.clinicalimaging.org/article/S0899-7071(24)00123-2/fulltext. Accessed 28 May 2024.
- Ramwala, Ojas A., et al. “Establishing a Validation Infrastructure for Imaging-Based AI Algorithms Prior to Clinical Implementation.” Journal of the American College of Radiology, Journal of the American College of Radiology, 21 May 2024, https://www.jacr.org/article/S1546-1440(24)00451-4/abstract. Accessed 26 May 2024.
- Nguyen, Derek L., et al. “Patient Characteristics Impact Performance of AI Algorithm in Interpreting Negative Screening Digital Breast Tomosynthesis Studies.” Radiological Society of North America, Radiological Society of North America, 21 May 2024, https://pubs.rsna.org/doi/10.1148/radiol.232286. Accessed 27 May 2024.
- Cheng, Wei. “AI in Radiology: 10 Use Cases, Benefits and Examples.” Itransition, 22 March 2024, https://www.itransition.com/ai/radiology. Accessed 25 May 2024.
- Morton, Will. “ChatGPT tested on cardiac imaging questions.” Aunt Minnie, 28 May 2024, https://www.auntminnie.com/imaging-informatics/artificial-intelligence/article/15673501/chatgpt-tested-on-cardiac-imaging-questions. Accessed 29 May 2024.
- “Radiology Advances First Issue Released.” RSNA, 14 May 2024, https://www.rsna.org/news/2024/may/radiology-advances-first-issue-released. Accessed 26 May 2024.
- AI from a Radiologist's Perspective. Performance by Jeff Chang, AWS Events, 2020. Youtube, https://www.youtube.com/watch?v=UM0tx5UKoSo. Accessed 25 May 2024.
- Sair, Haris. “Johns Hopkins Radiology Explores the Potential of AI in the Reading Room.” Johns Hopkins Medicine, https://www.hopkinsmedicine.org/news/articles/2023/11/johns-hopkins-radiology-explores-the-potential-of-ai-in-the-reading-room. Accessed 30 May 2024.
- Hogrebe, Anna. “Philips and Norwegian Vestre Viken Health Trust deploy AI-enabled clinical care to help radiologists improve patient care.” Philips.com, Philips.com, 20 November 2023, https://www.philips.com/a-w/about/news/archive/standard/news/articles/2023/20231120-philips-and-norwegian-vestre-viken-health-trust-deploy-ai-enabled-clinical-care-to-help-radiologists-improve-patient-care.html. Accessed 2 June 2024.